Advancing AI-Powered Orthopedic Image Segmentation: Mitigating Bias for Enhanced and Equitable Healthcare.
AI-powered segmentation of hip and knee bony anatomy has revolutionized orthopedics, transforming both pre-operative planning and post-operative assessment. However, despite the remarkable advancements in deep learning computer vision algorithms for medical image segmentation, a critical issue remains largely unexplored: the potential for biases inherent within these AI-powered models. This study addresses this critical concern by conducting a comprehensive re-examination of deep learning-driven hip and knee bony anatomy segmentation using plain radiographs, with a specific focus on identifying and addressing discernible biases related to sex and race. This study, through extensive evaluation, provides insights into the underlying causes of biases in AI-based knee and hip image segmentation. It presents targeted mitigation strategies to alleviate biases related to sex and race, fostering automatic segmentation results that are fair, impartial, and safe in the context of AI.The lessons derived from this research will be invaluable as AI progresses in healthcare, guiding the development of deep learning models that prioritize inclusivity, ethical practices, equity, and an unbiased healthcare landscape. We believe that our contribution holds the potential to enhance clinical outcomes, supporting both clinical decision-making and osteoarthritis (OA) research, thus we have made all codes and the dataset publicly and freely available for open scientific research and any educational purposes.
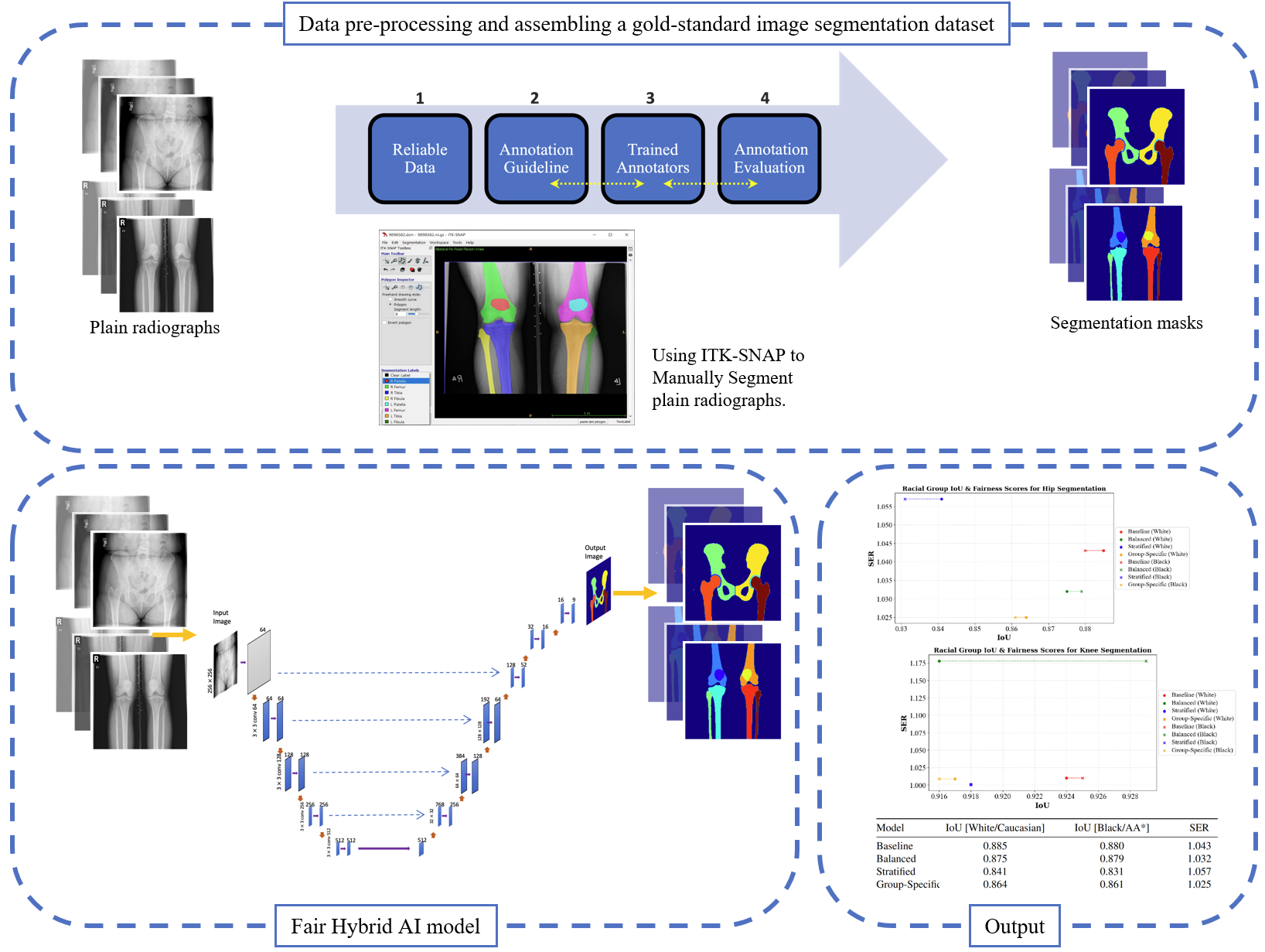